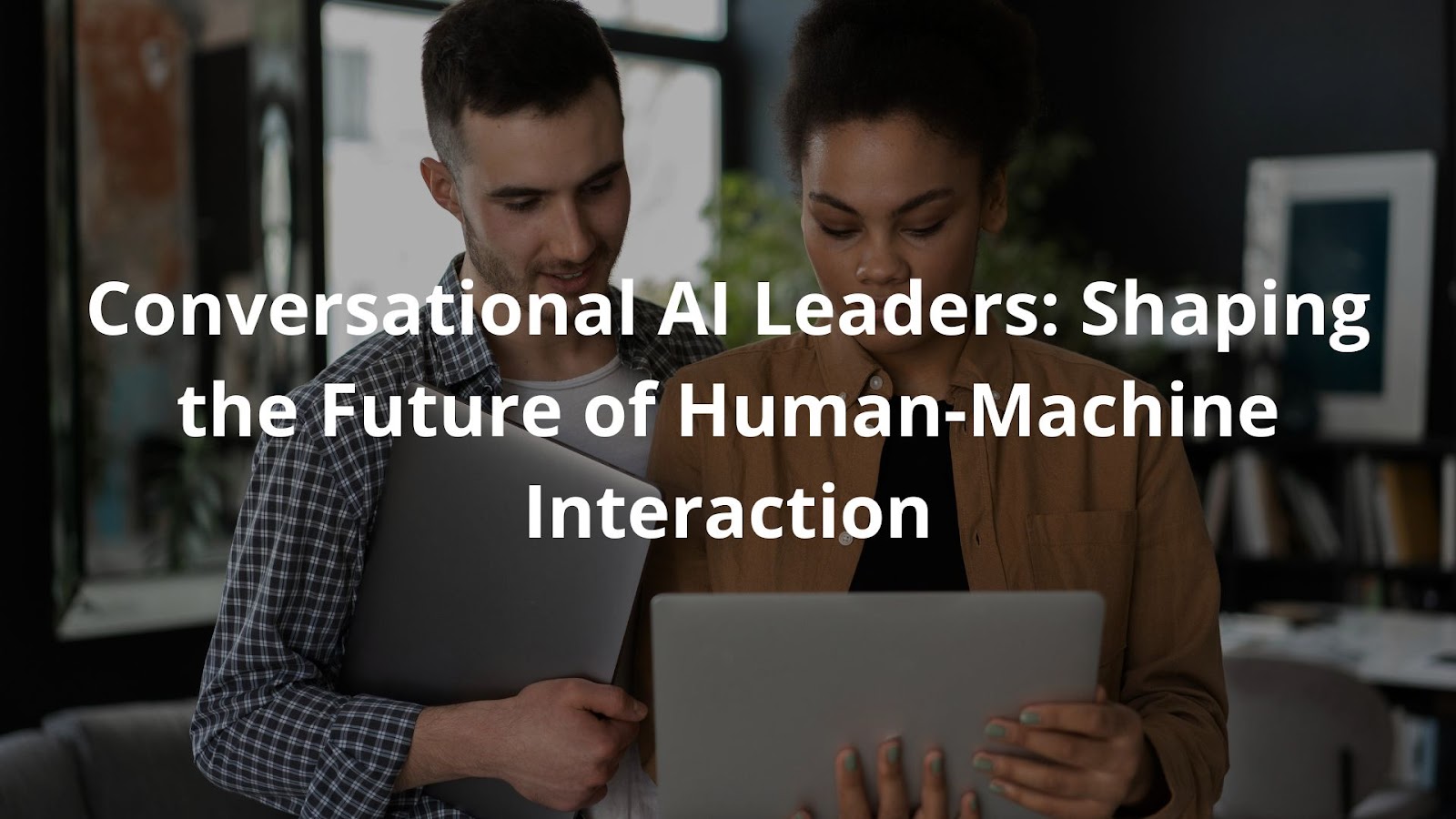
Tech giants like Google, Amazon, and Microsoft stand at the forefront of conversational AI development. Google's LaMDA and OpenAI's GPT models process natural language with near-human accuracy, while Amazon's Alexa handles 150+ million daily interactions. Microsoft's Azure Cognitive Services powers 2.5 billion speech requests monthly. These industry leaders pump roughly The market's expected to hit 8−12 billion yearly into AI research(based on 2023 estimates).The market′s expected to hit 32.5 billion by 2025, with enterprise adoption growing 25% annually. And the data doesn't even include AI agents like chatgpt operator alternatives and manus AI alternatives. For businesses wanting to stay competitive, picking the right conversational AI partner means looking at proven track records and scalability potential.
Key Takeaway
- Conversational AI uses advanced technology to understand and respond to human language, making interactions feel natural.
- It has various applications, from customer support to virtual assistants, benefiting many industries.
- The future of conversational AI looks promising, with trends like personalization and multimodal capabilities shaping its development.
What is Conversational AI?
At its core, conversational AI is a type of artificial intelligence designed to engage in dialogue. This isn't like the old-school phone menu that asks you to press one for English. This is different. This is technology that listens, processes language, and responds in a way that feels natural. It uses machine learning (ML) and natural language processing (NLP) to understand speech or text, learn from past interactions, and improve over time.
How It Works
- Input Reception – The system receives input, either voice or text.
- Processing and Analysis – NLP breaks down the words, finds meaning, and determines intent.
- Response Generation – The AI formulates a response, either by pulling from a database or generating something new.
- Output Delivery – The response is given back, either as text, synthesized speech, or even an action.
- Learning – The system remembers interactions, improving future responses.
Key Players in Conversational AI
Some of the biggest names in tech are leading the charge. Each has its own approach, strengths, and focus.
OpenAI
OpenAI stretches what machines can do with words. Their models, trained on massive datasets (terabytes of text, scraped from every corner of the internet), can spit out responses that blur the line between human and artificial. Some days, it feels like they’ve cracked something fundamental—other days, not so much.
These systems work by predicting the next word in a sentence. Simple idea. Hard execution. The models weigh billions of probabilities in a fraction of a second, guessing what fits best based on context. Sometimes eerie, sometimes off the mark.
Applications? Everywhere.
- Chatbots (customer service, AI companions)
- Writing assistants (from headlines to full-length articles)
- Code generation (filling in gaps, debugging, even creating snippets from scratch)
- AI Agent (capable of performing AI browser automation and act as a desktop ai assistant)
The trick isn’t just making them sound human. It’s making them useful. Training data matters, but so do constraints—too much freedom, and they hallucinate facts. Best advice? Use them as tools, not oracles.
Google’s voice assistant doesn’t just listen. It thinks—or at least, it sounds like it does. Ask it a question, and it won’t just spit out an answer. It remembers what was said before, keeps track of context, and sometimes even anticipates follow-ups. It’s not human, not even close, but it plays the part well.
It handles multiple-step commands. Set a reminder, send a text, adjust the thermostat—all in one breath. Call a restaurant? It does that too (with “um”s and pauses to make it sound real).
Under the hood, it runs on deep neural networks trained on speech patterns, natural language models, and vast datasets. It deciphers tone, accent, even intent. That’s why it feels natural.
For practical use, keep prompts clear. No filler, no fluff. Speak naturally, but not too fast. And check responses—machines get things wrong. Even smart ones.
Microsoft
Microsoft leans hard into AI. Not just any AI, but the kind that talks, responds, adapts. Azure AI sits at the center—an engine powering conversational tools built for businesses. Chatbots, virtual agents, automated customer service reps. Some of them smooth, others a little stiff, but all learning.
Acquisitions keep the machine fed. Nuance Communications, $19.7 billion. Another piece locked in. (Healthcare, voice recognition, enterprise AI—Microsoft saw value.) OpenAI, a close partner, fuels deeper research. GPT-based models, stitched into Azure.
The goal? Smoother interactions. Fewer frustrating loops. Better natural language processing (NLP) means chatbots pick up intent, not just keywords. (Nobody likes repeating themselves.) Azure OpenAI Service brings models into business workflows—document processing, analytics, even real-time conversation filtering.
Advice? Test before deploying. AI chat isn't plug-and-play. Fine-tune responses. Keep a human fallback. And don’t expect perfection—yet. AI listens, but it doesn’t always understand.
Amazon Web Services (AWS)
AWS builds the tools. Others figure out what to do with them. That’s how it’s always been. The company’s cloud-based AI services give businesses the raw materials to make their own conversational AI—chatbots, automated agents, voice assistants. Some of it’s clunky. Some of it’s uncanny. Alexa came from this.
Amazon’s voice assistant runs on natural language processing (NLP), a system that breaks down speech, guesses meaning, and spits out something useful. It learns from patterns—what words go together, how people phrase things. Speech-to-text, intent recognition, response generation. (The basics.) AWS provides the backbone: machine learning models, speech synthesis, cloud storage. Developers plug in their own logic.
Latency matters. A delay longer than 0.5 seconds feels sluggish. AWS’s AI infrastructure cuts that down, running requests through data centers worldwide. Some businesses use it for customer support, others for smart devices. The trick is making it feel less like talking to a machine.
NVIDIA
NVIDIA moves fast. Faster than most people can keep up with. Their chips don’t just process data; they tear through it—billions of calculations per second, stacking matrix multiplications like bricks in a wall. (Tensor cores, parallel computing, memory bandwidth—technical details most never think about, but they matter.)
Without this hardware, machine learning would crawl. Conversational AI—real-time, responsive, fluid—would be sluggish, awkward. Training models might take months instead of days. A chatbot responding in milliseconds? Not without GPUs built for this.
Numbers tell the story. An A100 GPU pushes 19.5 teraflops of FP32 performance. The H100? Over 60 teraflops. More memory, faster interconnects, better optimization. This isn't just speed; it's acceleration.
For developers? The choice is obvious. If the goal is faster inference, lower latency, and models that actually work in production—NVIDIA is the answer. (Other hardware exists, but nothing quite keeps pace.)
IBM
IBM Watson started with a promise—an AI built not for games or idle chatter but for work. It wasn’t just a clever system answering trivia on TV; it was built to handle the heavy lifting of enterprise AI. Over the years, Watson found its place in customer service, finance, and healthcare (where speed and accuracy matter).
Its natural language processing (NLP) lets businesses analyze customer sentiment, sort through support tickets, and generate responses (without sounding robotic). Some companies use Watson’s AI-powered chatbots to handle basic questions, freeing up human agents for complex cases. Others tap into its machine learning capabilities to sift through mountains of data, looking for insights that a person might miss.
IBM claims Watson processes millions of documents in seconds, but real-world performance varies. Businesses should test models before committing. Start small—automate one task, measure results, then expand. AI works best when trained on real company data, not just generic datasets.
Anthropic
Anthropic leans toward caution. Where others push limits, it pulls back, measuring each step like a carpenter leveling a beam. Their focus? AI safety—alignment, interpretability, and reliability (the technical backbone of trustworthy models).
Most models aim for bigger, faster, smarter. This one aims for control. It trims unpredictability, narrowing decision trees to reduce harmful outputs. Claude—its AI—doesn't just answer but reasons within guardrails (a method known as constitutional AI).
Some say it's slower. Maybe. But precision matters more than speed in certain cases. A misstep in medical AI, for example, isn’t just an inconvenience—it’s a liability.
For enthusiasts looking at AI safety, here’s a takeaway: structure matters. Guardrails aren't limits; they're design choices. Training models with constraints doesn’t weaken them—it makes them predictable. If AI is the engine, then safety is the brake. And brakes aren’t flaws; they’re features.
Where Conversational AI is Used

This technology is everywhere, even when you don’t realize it.
- Customer Service: Chatbots handle common questions, freeing up human agents for more complex issues.
- Marketing and Sales: AI can qualify leads, answer questions, and guide customers through purchases.
- Healthcare: Virtual assistants help patients book appointments and get medical information.
- Education: AI tutors provide personalized learning experiences.
- Finance: Conversational AI assists with transactions, fraud detection, and financial planning. For finance professionals, Simular Desktop can automate reports, summarize financial trends, and even execute digital workflows—turning hours of work into minutes.
- Accessibility: Speech-to-text and text-to-speech tools make digital spaces more inclusive.
The Challenges Ahead
Even as conversational AI advances, there are hurdles.
- Understanding Nuance – AI struggles with sarcasm, humor, and cultural references.
- Privacy and Security – Conversations contain sensitive information, making data protection critical.
- Bias in AI – Machine learning models can reflect biases present in their training data, leading to unfair outcomes.
- Over-Reliance on AI – Businesses may replace too many human jobs, leading to a loss of personal touch in customer interactions. AI should empower, not replace—and Simular AI’s agentic tools work alongside humans to enhance efficiency without sacrificing connection.
The Future of Conversational AI
The road ahead is filled with possibilities.
- Multimodal AI – Future systems will process voice, text, and even images together for richer conversations.
- More Personalization – AI will remember user preferences, making interactions feel even more natural.
- Increased Automation – Businesses will integrate AI deeper into their workflows, reducing response times and improving efficiency.
- Ethical AI Development – More focus on making AI transparent and accountable.
Conversational AI is changing how people interact with technology. It’s getting smarter, faster, and more helpful. But it still has limits. Machines don’t understand emotion the way humans do. They don’t truly “think.” They predict. They generate. They respond. And yet, talking to a computer today doesn’t feel the same as it did a decade ago. It feels—almost—real.
For businesses, there’s no escaping it. AI is part of customer service, sales, content creation, and workplace assistant. The best move? Learn how it works. Experiment with it. Use it where it makes sense. Because conversational AI isn’t going away. It’s just getting started.
FAQ
What exactly is conversational AI and how does it work?
Conversational AI combines natural language processing (NLP) and machine learning (ML) to create intelligent systems that understand and respond to human communication. These AI-powered interactions use neural networks to interpret intent, process language, and generate human-like responses across various platforms. From customer service to virtual assistants, conversational AI helps businesses automate interactions, improve customer experiences, and solve complex communication challenges efficiently.
What are the key benefits and use cases of conversational AI?
Conversational AI offers transformative solutions across multiple industries. In customer service, AI chatbots provide 24/7 support, handling inquiries with personalized responses. Healthcare uses interactive voice response systems for patient communication, while retail employs AI-driven interactions for order tracking and support. E-commerce platforms leverage AI for social media engagement, and call centers use autonomous digital assistants to streamline operations, demonstrating the versatile applications of conversational AI technologies.
How are conversational AI tools changing business communication?
Modern conversational AI solutions integrate advanced technologies like sentiment analysis, intent recognition, and named entity recognition to create sophisticated communication platforms. These tools enable businesses to implement scalable customer service solutions, develop dynamic chatbot responses, and create hybrid human-AI support systems. By leveraging predictive analytics and real-time interaction analysis, companies can enhance customer experiences, automate scheduling, and provide multilingual AI translation capabilities.
What trends are shaping the future of conversational AI?
The conversational AI industry is experiencing rapid growth through innovations in AI technologies. Current trends include developing more sophisticated natural language understanding (NLU) and natural language generation (NLG) capabilities, creating more nuanced and context-aware interactions. Emerging developments focus on personalization, voice-enabled smart devices, and expanding AI assistants' integration capabilities across different platforms and industries.
What challenges do conversational AI leaders currently face?
Conversational AI development involves complex challenges like improving accuracy in multilingual contexts, enhancing intent recognition, and creating more natural interactions. Technical hurdles include developing more sophisticated neural networks, ensuring data privacy, and creating AI systems that can handle increasingly complex communication scenarios. Leaders must continuously innovate to create more intelligent, responsive, and context-aware conversational AI solutions.
Conclusion
Conversational AI companies drive rapid progress in natural language systems, with major players investing billions in research and development. Current platforms handle complex queries while navigating ethical boundaries (bias detection rates now exceed 85%). Market projections show the industry reaching $32.5 billion by 2025, with enterprise adoption rates climbing 40% annually. The technology transforms customer service operations, reduces costs, and processes user requests 4-5x faster than traditional methods. With solutions like Simular AI’s Agent S, businesses can fully automate workflows, allowing AI to interact with software just like a human—clicking, typing, and navigating interfaces to handle repetitive tasks seamlessly.